Summary
- Pathogenetic small-variant detection in the PMS2 gene related to Lynch syndrome is confounded by the pseudogene PMS2CL.
- DRAGEN 4.3 introduces a refined algorithm for empowering small-variant detection in PMS2 using whole-genome sequencing.
- By applying this approach on 22 non-cell-line samples, all expected P/LP variants are detected.
- This method is extensible to other genes with single- or multi-copy paralogues.
Pathogenetic variant detection in PMS2 for Lynch syndrome screening is confounded by the pseudogene PMS2CL
Each of our trillions of cells contains a complete set of DNA that itself contains billions of subunits. How is it physically possible to create and maintain it all? An important part of the answer is that our genomes contain a wide array of error-correcting mechanisms. One of these mechanisms is called mismatch repair (MMR), which recognizes and fixes DNA that isn’t paired up properly. An example would be if an A is opposite a G instead of a T.
When someone has a defect in an MMR gene, they have Lynch syndrome, which is the second most common form of genetic predisposition to cancer.1 The combined prevalence of pathogenic variants across the four major Lynch syndrome genes (MSH2, MSH6, MLH1, and PMS2) could be as high as 1 in 279 in the general population.2 Given the high rates of colorectal cancer, uterine cancer, and other forms of malignancies in people with Lynch syndrome and the importance of early detection, accurate genetic screening has the tremendous potential to improve health outcomes.
PMS2 poses particular challenges for genetic screening of Lynch syndrome due to the presence of a pseudogene, PMS2CL. The high similarity between the two regions (Figure 1) creates significant challenges for variant calling.3 Misalignment and ambiguous mapping of reads originating from this region can lead to false-positive or false-negative results, impacting clinical decision-making and patient care.4 Currently available methods may require dedicated single-gene wet lab tests, such as long-range PCR, which are difficult to scale.
We aim to address these challenges and improve the reliability of small-variant calling in PMS2 exon 11–15 using Illumina’s popular PCR-free whole-genome sequencing assay.
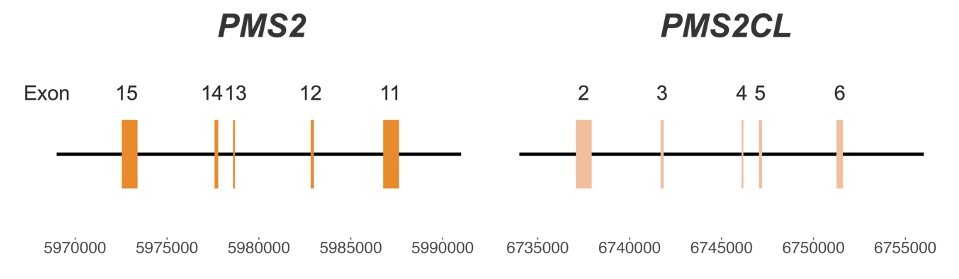
Figure 1: High-homology region between PMS2 and PMS2CL genes—Coordinates are from hg38.
Multi-region joint detection approach
Conventional variant callers rely on an aligner to first uniquely map sequence reads to their original genomic location before checking for small differences. This method works well when the read or read pairs resemble only one part of the reference genome, but it struggles when two or more regions match equally well. Since at least 5% of the genome has one or more near-identical copies, the true origin of a read is often uncertain. If a group of reads is mapped with low confidence, a conventional variant caller might ignore the reads, even though they contain useful information. If a read is mismapped (that is, if the primary alignment is not the true source of the read), it can result in variant detection errors.
To address these challenges, we developed a novel computational method called multi-region joint detection (MRJD). Instead of considering each region in isolation and genotyping them individually, MRJD considers all locations from which a group of reads may have originated and attempts to detect the underlying sequences jointly. This approach retains reads with ambiguous alignment and is apt for instances of read misalignment due to gene conversion or crossover events.
Figure 2 shows the general workflow of MRJD using PMS2 and PMS2CL high-homology regions as an example. In short, MRJD takes primary alignments in all paralogous regions (regardless of mapping quality), builds and places all haplotypes based on reads and prior knowledge, and computes joint genotypes to call small variants.
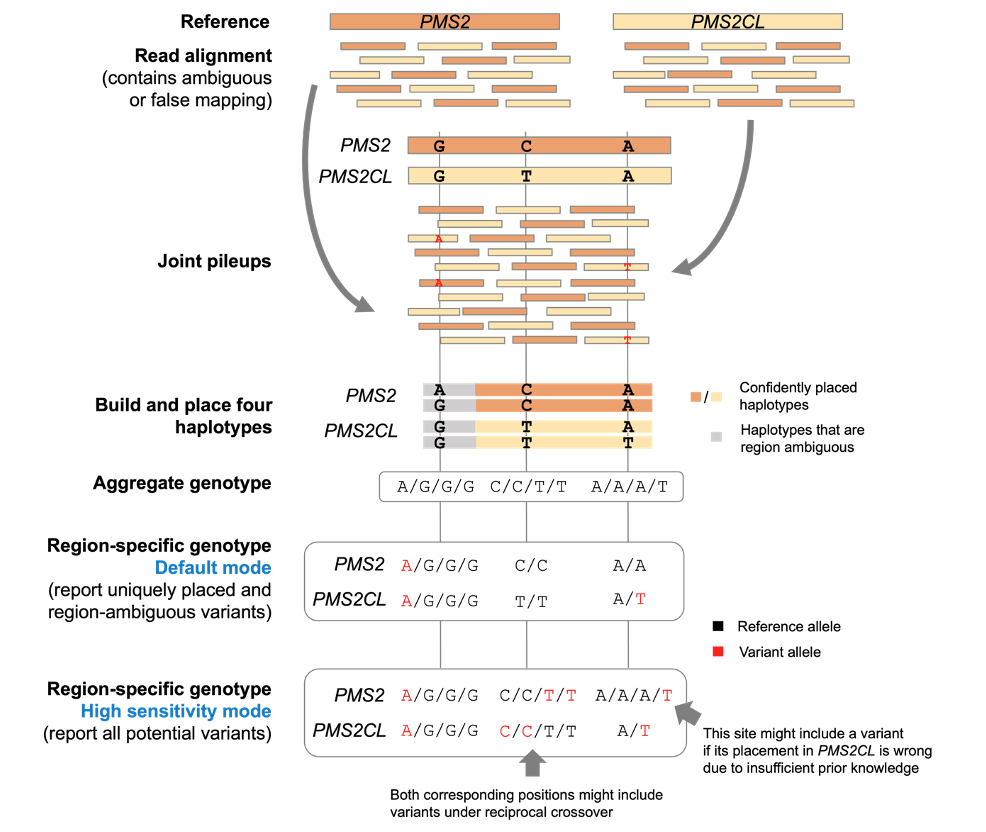
Figure 2: MRJD workflow.
MRJD high sensitivity mode is recommended for applications where sensitivity is paramount
As shown in Figure 2, there are two modes of MRJD: a default mode that provides balance between precision and recall, and a high sensitivity mode that provides maximum recall at the expense of precision. In settings where identification of all potentially pathogenic variants is paramount and orthogonal confirmation of the placement of such variants is viable (for example, long-range PCR-based tests), we recommend using MRJD high sensitivity mode.
MRJD performance on cell line samples
We benchmarked MRJD's variant calling performance in the PMS2 high-homology region from 147 cell line samples from the Illumina Polaris 1 diversity panel.5 Ground truth was established using orthogonal small variant calls derived from long-range PCR data.5 MRJD high sensitivity mode achieves substantially higher recall compared to the DRAGEN small variant caller, with aggregated recall around 99.7% and 97.1% for SNVs and indels, respectively (Figure 3). The lower recall for indels could be due to the higher error rate for indels compared to SNVs in long-range PCR data. To address this issue, we conducted concordance analysis with the long-read-based approach,6 utilizing an independent dataset comprising 147 cell line samples representing diverse ancestries from the 1000 Genomes Project. The aggregated recall against the long-read-based approach is greater than 99.7% for both SNVs and indels.
The high recall rate from MRJD high sensitivity mode is achieved by placing all possible variants in all paralogous regions, which comes at the cost of lower precision. To measure the spurious call rate, we compared merged orthogonal variant calls in both PMS2 and PMS2CL against MRJD High Sensitivity mode calls in PMS2 only. This analysis shows that the spurious call rate is lower than 0.7%, which means that nearly all the reported alleles do indeed occur in the samples but are reported in both locations by MRJD high sensitivity (ambiguous placement).
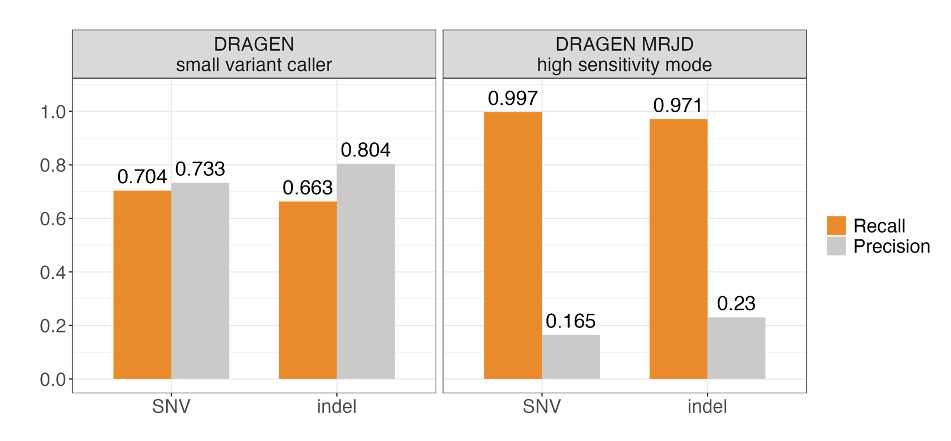
Figure 3. Aggregated SNV and indel performance between DRAGEN small variant caller (DRAGEN VC) and MRJD high sensitivity mode on 147 samples from the Illumina Polaris 1 diversity panel—Orthogonal small variant calls were derived from long-range PCR data.5 RTG Tools ploidy-squash mode is used to generate benchmark statistics. All perfect homopolymers > 6 bp and imperfect homopolymers > 10 bp have been excluded from the analysis.
MRJD performance on non-cell-line samples
To assess how the MRJD approach would perform in a real-world setting, we collaborated with Broad Clinical Labs and Tempus AI to evaluate MRJD performance on a total of 22 non-cell-line samples, 16 of which have potential clinically relevant variants in PMS2 and PMS2CL high homology regions (11 from Broad Clinical Labs, 5 from Tempus AI). An additional 6 samples from Broad with known pathogenic variants associated with nemaline myopathy occurring in the triplicate region of the NEB gene were also included in the analysis, since this region is another segmental duplication covered by MRJD in DRAGEN v4.3.
MRJD high sensitivity mode was able to detect the presence of all expected clinically relevant small variants in all 22 samples (Table 1).
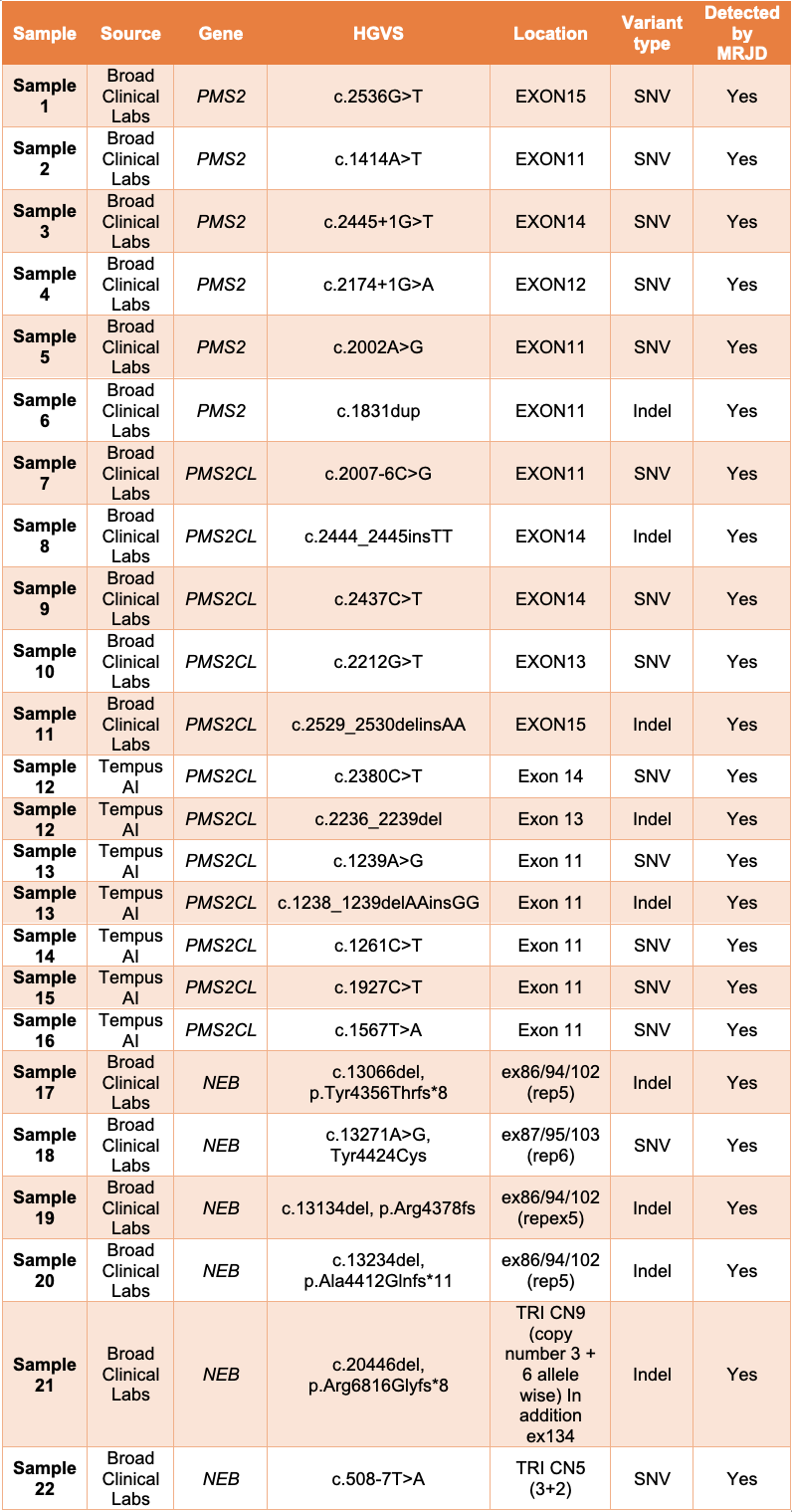
Table 1: MRJD high sensitivity mode results on 22 non-cell-line samples with potential clinically relevant variants—All listed variants were orthogonally confirmed and determined to be in either PMS2, PMS2CL, or NEB. Results are provided by Broad Clinical Labs and Tempus AI.
Estimate P/LP variant call rate in presumed unaffected samples
An efficient screen-and-confirm reflex approach requires an overall low positive rate in samples from unaffected individuals. To estimate this rate, we measured the P/LP variant call rate using 147 cell lines from the 1000 Genomes Project. The P/LP variant call rate in the PMS2 gene is 1/147 (0.68%), indicating low reflex burden in samples from unaffected individuals.
Discussion
In summary, we introduce here a novel computational strategy, multi-region joint detection, that addresses the challenge of de novo germline small variant calling in paralogous regions using WGS data, achieving improved sensitivity and specificity. By applying this approach on PMS2 and NEB genes, we demonstrate that this work contributes to a more reliable detection of variants associated with Lynch syndrome and with nemaline myopathy, enabling better risk assessment and personalized management strategies for affected individuals.
MRJD is designed to be a versatile framework that works for paralogous regions with high sequence identity. In addition to PMS2 and NEB, MRJD included in DRAGEN v4.3 also supports germline small variant calling in the repetitive regions of five other clinically relevant and challenging genes: SMN1, SMN2, STRC, IKBKG, and TTN. It is estimated that the human genome contains 200–500 medically relevant genes with problematic regions, where high homology is a primary concern.7-8 We anticipate that our approach will pave the way for further research on variant calling in other medically relevant genes that face similar homology challenges.
Supplementary materials
In addition to the results presented in this blog, we also collaborated with Tempus AI to evaluate the MRJD caller using 150 cell line samples. The findings from this collaboration were presented at the ISMB 2023 conference. For detailed insights, refer to the publication available at this link. It's worth noting that the performance of the MRJD caller has seen further improvements since the publication of this abstract.
Availability
The software is available in the v4.3 release of DRAGEN, for which installation files and release notes are available here. For more information on the MRJD caller, refer to the user guide here or contact ffg-info@illumina.com.
For more information or a DRAGEN trial license for academic use, please contact dragen-info@illumina.com.
Acknowledgement
We want to thank our collaborators Marina DiStefano and Edyta Malolepsza from Broad Clinical Labs, and Francisco M. De La Vega and Pavana Anur from Tempus AI, for assessing the MRJD caller on non-cell-line samples.
References
- Lynch HT, Lynch PM, Lanspa SJ, Snyder CL, Lynch JF, Boland CR. Review of the Lynch syndrome: history, molecular genetics, screening, differential diagnosis, and medicolegal ramifications. Clin Genet. 2009;76(1):1-18. doi:10.1111/j.1399-0004.2009.01230.x
- Win AK, Jenkins MA, Dowty JG, et al. Prevalence and Penetrance of Major Genes and Polygenes for Colorectal Cancer." Cancer Epidemiol Biomarkers Prev. 2017;26(3):404-412. doi:10.1158/1055-9965.EPI-16-0693
- van der Klift HM, Mensenkamp AR, Drost M, et al. Comprehensive Mutation Analysis of PMS2 in a Large Cohort of Probands Suspected of Lynch Syndrome or Constitutional Mismatch Repair Deficiency Syndrome. Hum Mutat. 2016;37(11):1162-1179. doi:10.1002/humu.23052
- Huang KL, Mashl RJ, Wu Y, et al. Pathogenic Germline Variants in 10,389 Adult Cancers. Cell. 2018;173(2):355-370.e14. doi:10.1016/j.cell.2018.03.039
- Gould GM, Gauman PV, Theilmann MR, et al. Detecting clinically actionable variants in the 3′ exons of PMS2 via a reflex workflow based on equivalent hybrid capture of the gene and its pseudogene. BMC Med Genet. 2018;19(1):176. doi:10.1186/s12881-018-0691-9
- Chen X, Harting J, Farrow E, et al. Comprehensive SMN1 and SMN2 profiling for spinal muscular atrophy analysis using long-read PacBio HiFi sequencing. Am J Hum Genet. 2023;110(2):240-250. doi:10.1016/j.ajhg.2023.01.001
- Ebbert MTW, Jensen TD, Jansen-West K, et al. Systematic analysis of dark and camouflaged genes reveals disease-relevant genes hiding in plain sight. Genome Biol. 2019;20(1):97. doi:10.1186/s13059-019-1707-2
- Wagner J, Olson ND, Harris L, et al. Curated variation benchmarks for challenging medically relevant autosomal genes. Nat Biotechnol. 2022;40(5):672-680. doi:10.1038/s41587-021-01158-1